“Keep reading” — A lack of feedback specificity is a pervasive issue in medical education, with each of us having received this feedback at some point during our training. As long-time sports fans, we clinician educators cannot help but compare our profession to the world of professional sports, where data and analytics are now routinely used to guide, prepare, and coach athletes such as Team Canada for the Olympic Games. With the emergence of competency-based medical education, data are more important than ever to provide information about trainees’ competency development. Notably, there is a strong association between the quality of training during residency and the resulting patient outcomes of graduates.
To enhance the richness of trainee feedback, medical education scholars have noted the unharnessed potential of clinical data in electronic health records (EHRs). A recent review developed a framework for how EHR-data could be leveraged through digital learning cycles to improve trainee performance. The three key steps included: (1) Attribution — Linking patients to the trainees who provided care, (2) Visualization — Displaying personalized data in an interpretable manner, and (3) Improvement — Using data to improve performance, guided through coaching and feedback.
The General Internal Medicine Inpatient Initiative Medical Education Database (GEMINI MedED) initiative at the University of Toronto aims to develop a big data program for medical education where trainees can access personalized EHR-data about their clinical care for learning, feedback, and assessment. We are tremendously grateful for the support of numerous organizations in this work including: GEMINI, University of Toronto, Harvard Medical School, Sinai Health System, PSI Foundation, Ontario Medical Association, and the Royal College of Physicians and Surgeons of Canada.
Attribution
Given the team-based and supervisory models inherent to medical education, attribution of clinical outcomes to individual trainees is a key consideration in using EHR data. To address this, the concept of resident-sensitive quality measures was developed, with measures focusing on outcomes that are mostly attributable to individual residents. For example, process measures, such as the percentage of a resident’s patient panel with diabetes prescribed a particular evidence-based treatment, are more attributable to individual residents’ care. This contrasts with outcome measures, such as the percentage of those same patients with diabetes whose HbA1c levels are at target, which is significantly influenced by factors outside of the residents’ control.
In the internal medicine context, our team has identified overnight call as a period when senior residents at our institution work with a high degree of independence, without immediate attending physician input. Therefore, we developed the GEMINI MedED database, linking 10 years of senior internal medicine resident call schedules to the patients they admitted during overnight internal medicine call. Clinical data was drawn from the existing GEMINI database, which has been used in numerous large scale epidemiological studies and has been shown to accurately track patient outcomes. Therefore, GEMINI MedED serves as a valuable ecosystem to retrospectively study resident-sensitive quality measures to answer questions such as how to select ideal performance metrics and interpret the observed variation.
Visualization
Dashboards have emerged as an effective approach to compile performance data and communicate this information to trainees. Prior studies have examined best practices in dashboard design, with key enablers including normative peer data, interactive visualization, and engagement with the end users during the design process. The GEMINI group has previously developed a dashboard platform in which attending internal medicine physicians across Ontario, Canada receive personalized reports on their clinical outcomes. Data is also referenced to peers at the same hospital to allow for team-based reflective practice. For GEMINI MedED, we plan to explore how existing infrastructure from GEMINI can be used to provide similar feedback for residents to guide reflective changes in practice. As an example, view a sample internal medicine physician feedback report from Ontario Health.
Improvement
Large scale reviews of audit-and-feedback approaches demonstrate that simply providing performance data is insufficient to change practice – meaningful engagement with the feedback is critical.
Prior work suggests that EHR-data can be viewed within Kolb’s model of experiential learning in which data is reflected upon through the support of a faculty coach, conceptualized to make connections with new knowledge, and integrated through the development of a personalized work plan. In the future, the GEMINI MedED team aspires to equip residents with personalized EHR-data to help them develop individualized work plans. Potential goals could include tailoring residents’ electives to optimize their case mix exposure or making changes to their clinical practice, such as an increased focus on resource stewardship.
Limitations
Although we are optimistic about the potential of EHR data, there are important technical and conceptual limitations to acknowledge. Technical limitations include evolving data science capabilities, such as a reliance on retrospective reporting of outcomes. Ultimately, real time data reporting has been described as a key enabler to maximize the utility of resident performance data. Conceptually, EHR data can be considered a type of “found data” which is repurposed for another use. Found data is often incomplete for its new goal and may have systematic bias related to how and why the data were initially collected. For example, using EHR data could accentuate a focus on easily measurable resident competencies such as resource stewardship, while inadvertently deemphasizing other important aspects of medical training such as communication skills. Therefore, we believe EHR data must be considered in context of programmatic assessment, or how it can contribute to existing methods of assessment to shape a comprehensive portfolio of assessment.
Summary
To realize our vision of building a big data program for medical education, careful attention is required to attribute care to trainees, visualize data, and catalyze improvement through coaching. GEMINI MedED serves as a foundational data platform through which we can begin answering these questions. Conceptualizing this program of research was the focus of a recent scholarly project in the Harvard Macy Institute Program for Educators in Health Professions and we are grateful to the program leadership and community for their feedback and support. GEMINI MedED is optimistic that digital learning will represent a new era in medical education assessment, akin to how big data revolutionized the world of professional sports.
Acknowledgements
The GEMINI MedEd team is comprised of multiple scholars, including Drs. Fahad Razak, Amol Verma, Martin Pusic, and Kulamakan (Mahan) Kulasegaram. We are grateful for their support and feedback in guiding this scholarly work.
Brandon Tang MD, MMSc is an Academic General Internist and Assistant Professor of Medicine at the University of Toronto. His areas of professional interest include big data, electronic health data, and competency-based medical education. He can be followed on LinkedIn.
Andrew CL Lam, MD is an Internal Medicine resident at the University of Toronto. His areas of professional interest include big data and medical oncology. He can be contacted via email.
Brian Wong, MD is a general internist and Professor of Medicine at the University of Toronto. Brian’s areas of professional interest include quality improvement and resource stewardship. He can be followed on LinkedIn.
Shiphra Ginsburg, MD, MEd, PhD, is an Education Clinician Scientist, internist, and Professor of Medicine at the University of Toronto. Her areas of professional interest include work-place based assessment, professionalism, and qualitative research. She can be followed on LinkedIn.
HMI Staff
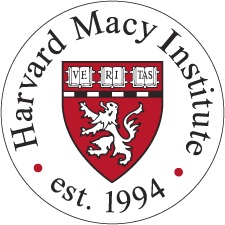